In fraud detection, machine learning algorithms can be used to analyze patterns in data such as credit card transactions, insurance claims, and tax returns, and identify anomalies that may indicate fraudulent activity.
For example, a credit card company might use machine learning to analyze patterns in its customers’ spending habits and flag any transactions that deviate significantly from the norm. The company could then investigate these flagged transactions to determine whether they are legitimate or fraudulent.
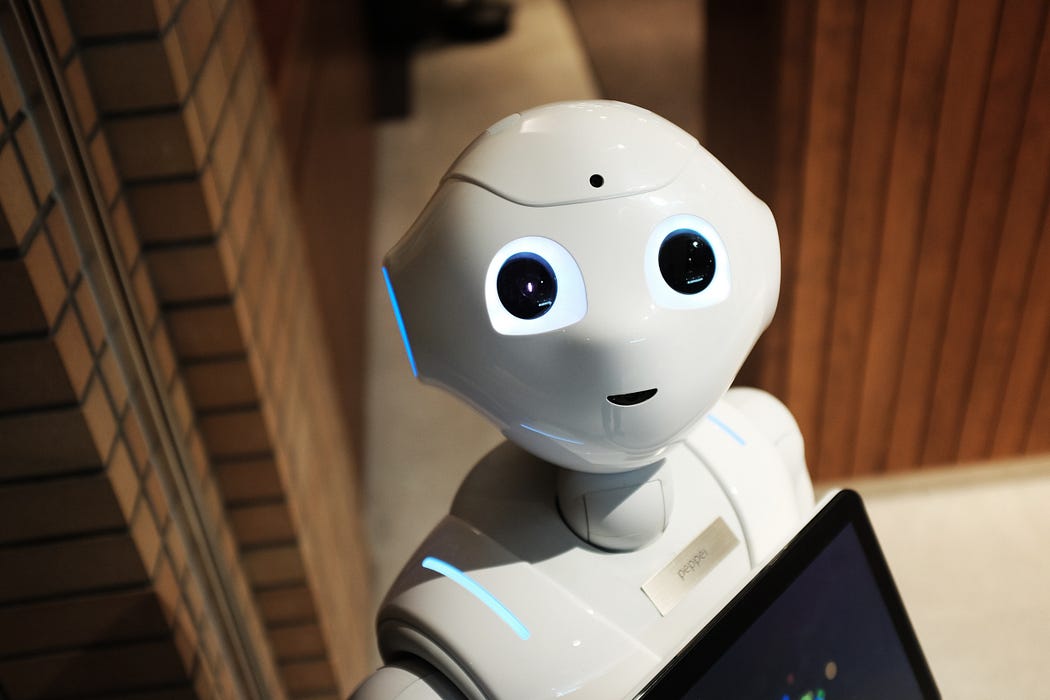
To train a machine learning model for fraud detection, the model would be fed a large dataset of labeled transactions, where the labels indicate whether a transaction is fraudulent or not. The model would then learn to identify patterns in the data that are associated with fraudulent activity.
Once the model is trained, it can be used to classify new, unseen transactions as either fraudulent or legitimate. If the model is accurate, it can help the credit card company catch fraudulent activity before it causes significant harm.
Here is a general outline of how you might implement a machine learning project for fraud detection:
- Define the problem and determine the goal of your model. In this case, the goal is to detect fraudulent activity in credit card transactions, insurance claims, or tax returns.
- Collect and clean the data that you will use to train the model. This could include transaction data, customer demographics, and other relevant information.
- Choose an appropriate model and evaluation metric. There are many different models that could be used for fraud detection, such as decision trees, random forests, and support vector machines. You will need to choose the model that works best for your data.
- Split the data into a training set and a test set. Use the training set to train the model, and the test set to evaluate its performance.
- Train the model on the training data. Fine-tune the model by adjusting the hyperparameters and adding regularization if necessary.
- Evaluate the model on the test set. Calculate the model’s accuracy, precision, and recall seeing how well it is performing.
- If the model performs well, deploy it in production. Monitor the model’s performance over time to ensure that it is still effective at detecting fraudulent activity.
This is just a high-level overview of the process, and there may be additional steps involved depending on the specific requirements of your project.
There are several machine learning algorithms that can be used for fraud detection, including:
- Decision trees: Decision trees are simple, easy-to-interpret models that can be used to classify transactions as either fraudulent or legitimate.
- Random forests: Random forests are a type of decision tree that uses an ensemble of decision trees to make predictions. They are more accurate than individual decision trees, but may be harder to interpret.
- Support vector machines (SVMs): SVMs are a type of model that can be used for binary classification tasks, such as fraud detection. They work by finding the hyperplane in a high-dimensional space that maximally separates the two classes.
- Neural networks: Neural networks are a type of model that can be used for a wide range of tasks, including fraud detection. They are composed of multiple layers of interconnected nodes and are trained using large amounts of data and powerful computational resources.
It’s worth noting that no single machine learning algorithm is the best choice for every problem, so you will need to experiment with different algorithms to see which one works best for your particular dataset and use case.
There are many machine learning software platforms that can be used for fraud detection, including:
- Python: Python is a popular programming language for machine learning and data science. It has a number of powerful libraries for machine learning, including scikit-learn, TensorFlow, and PyTorch.
- R: R is a programming language and software environment for statistical computing and graphics. It has a number of libraries for machine learning, such as caret and random Forest.
- WEKA: WEKA is a machine learning software platform developed at the University of Waikato in New Zealand. It includes a wide range of machine learning algorithms and is designed to be easy to use.
- IBM SPSS Modeler: IBM SPSS Modeler is a commercial software platform for data mining and predictive analytics. It includes a number of machine learning algorithms and is designed to be user-friendly.
- RapidMiner: RapidMiner is a commercial machine learning platform that includes a wide range of algorithms and is designed to be easy to use.
These are just a few examples of the many software platforms that can be used for fraud detection. The choice of software will depend on your specific needs and the resources available to you.
Here are a few ideas for machine learning mini projects in the area of fraud detection:
- Credit card fraud detection: Develop a machine learning model to detect fraudulent credit card transactions. You could use a dataset of labeled credit card transactions and try different algorithms to see which one performs best.
- Insurance fraud detection: Develop a machine learning model to detect fraudulent insurance claims. You could use a dataset of labeled insurance claims and try different algorithms to see which one performs best.
- Email spam detection: Develop a machine learning model to classify emails as spam or not spam. You could use a dataset of labeled emails and try different algorithms to see which one performs best.
- Fraudulent online reviews detection: Develop a machine learning model to detect fraudulent online reviews. You could use a dataset of labeled reviews and try different algorithms to see which one performs best.
- Fraudulent online transactions detection: Develop a machine learning model to detect fraudulent online transactions. You could use a dataset of labeled online transactions and try different algorithms to see which one performs best.
These are just a few ideas for machine learning mini projects in the area of fraud detection. The specific problem that you choose will depend on your interests and the resources available to you.